Context
Red Hat OpenShift Data Science started as an open source project called Open Data Hub. Its purpose was to simplify and focus the large number of open source tools available for creating and deploying AI and ML models on kubernetes. Red Hat had a large reputation in the open source community, but not in data science or the AI/ML product area at the time, so this was their first foray into the data science and AI community.
Understanding the Product Space
When I joined the product it was in its infancy and didn't have a UI yet. My first task was to figure out who the product's users would be, and make sure we understood their needs and use cases. I dove into understanding the basics of data science and the tools data scientists use, and had a countless number of informal conversations with existing product and engineering team members and potential users to hear their viewpoints and gather their plans for the growth of the product.
I ran a mini-workshop to get the highest-level stakeholders' opinions documented, and make sure they had a shared understanding of a problem statement for the product, who our users are, and future product goals.
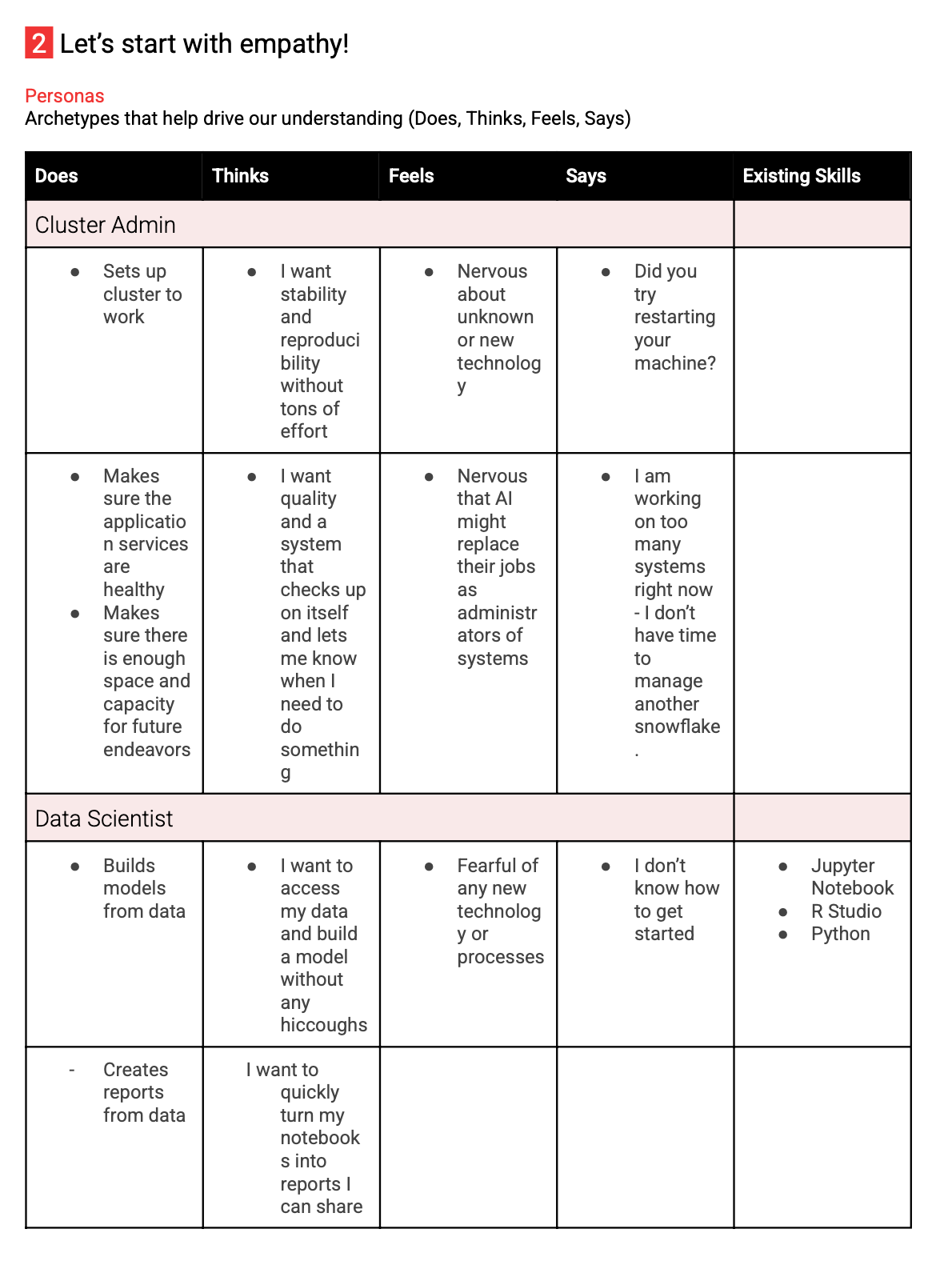
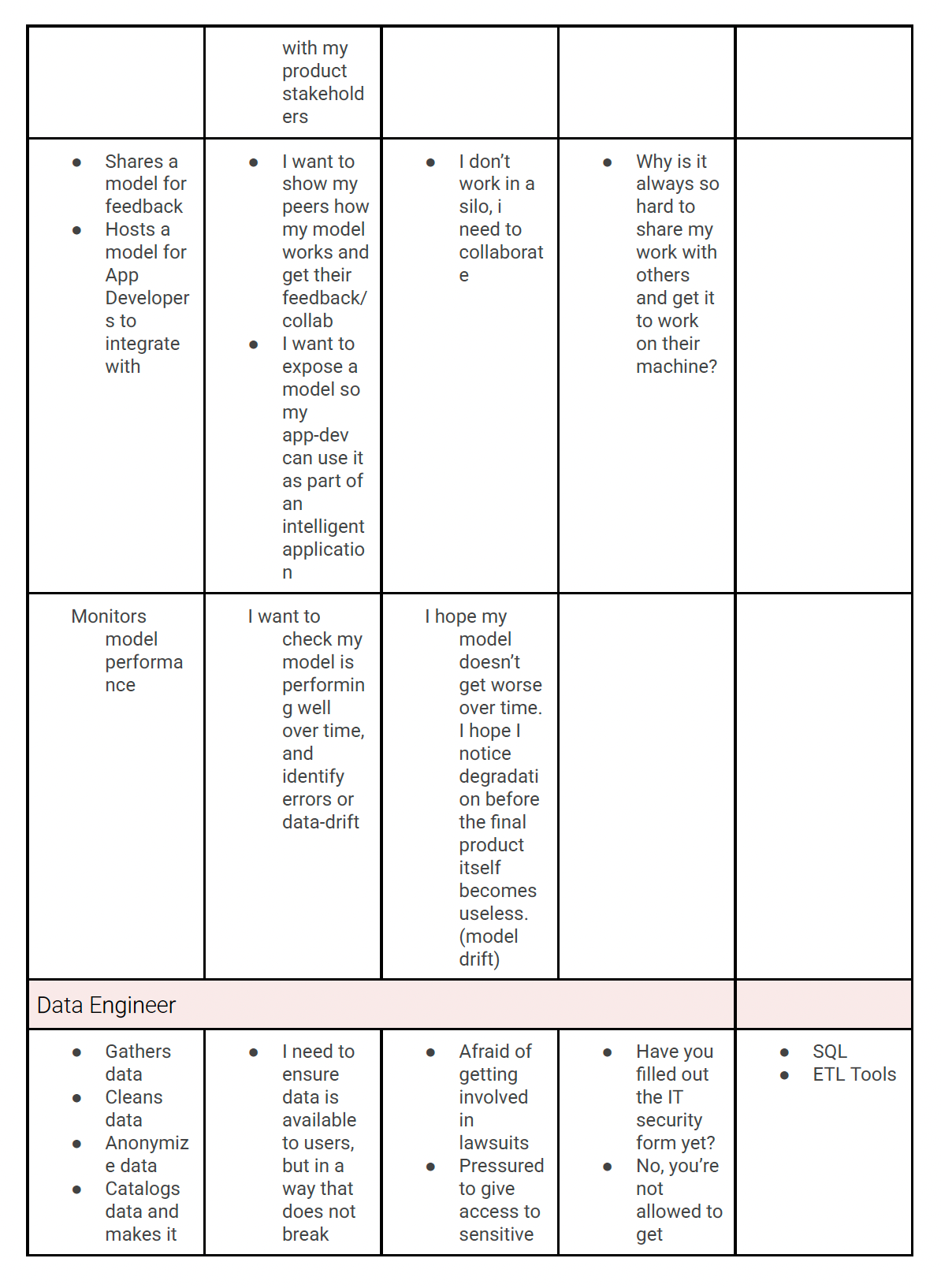
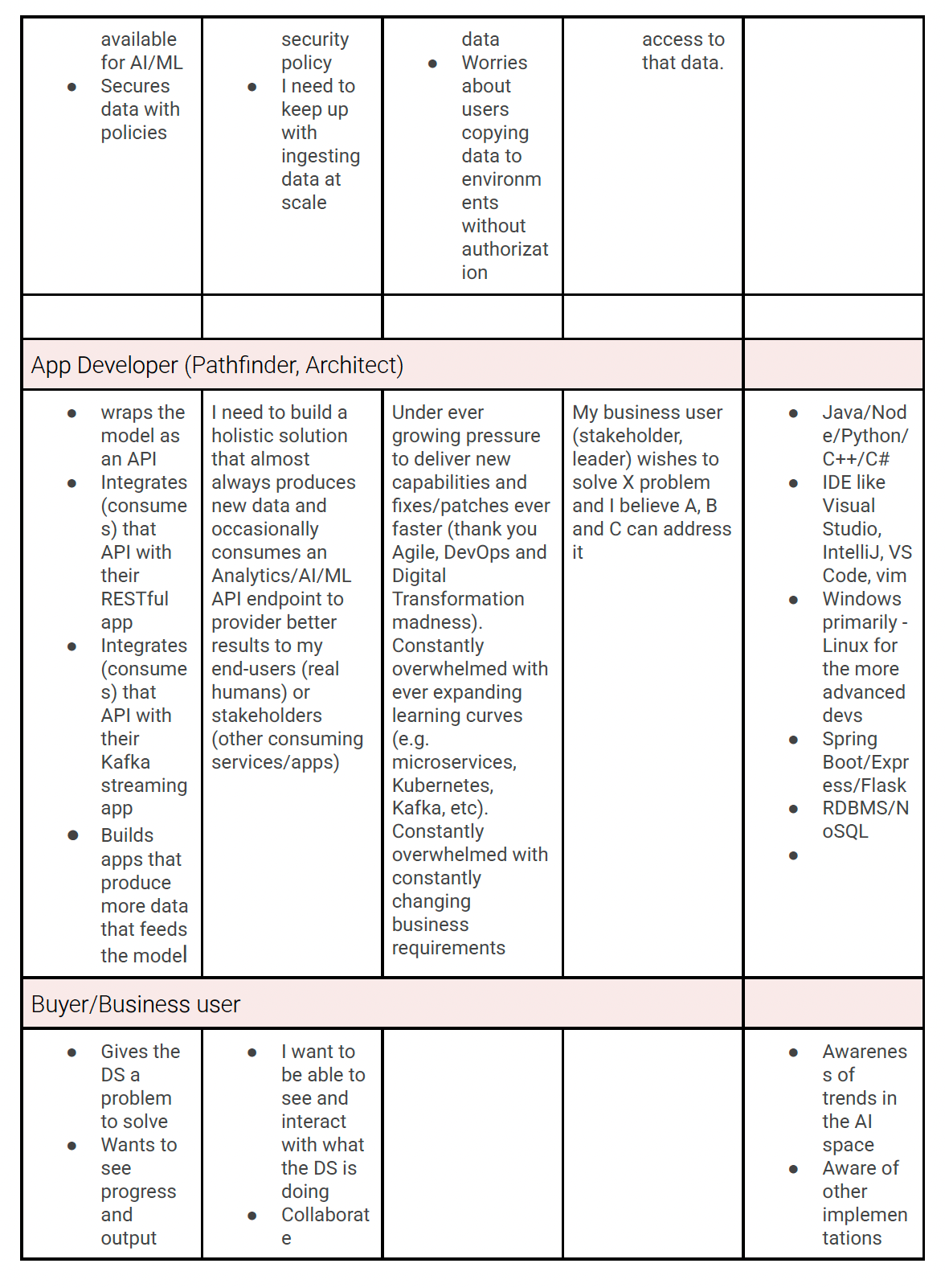
With this information in hand I mapped the high-level needs of each user persona, and working with the stakeholders in weekly meetings, created a journey map to understand which users were expected to do which parts of the process.
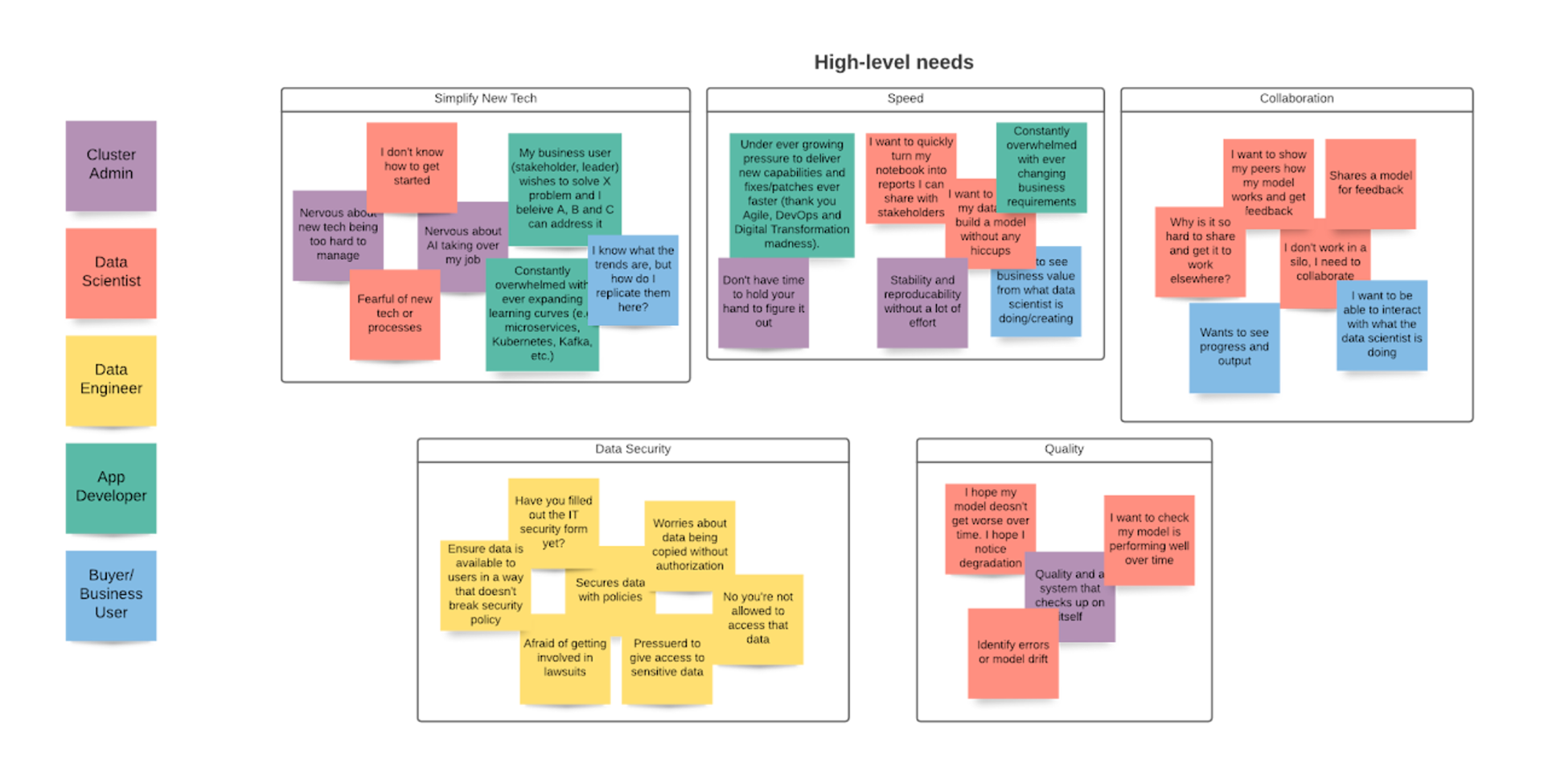
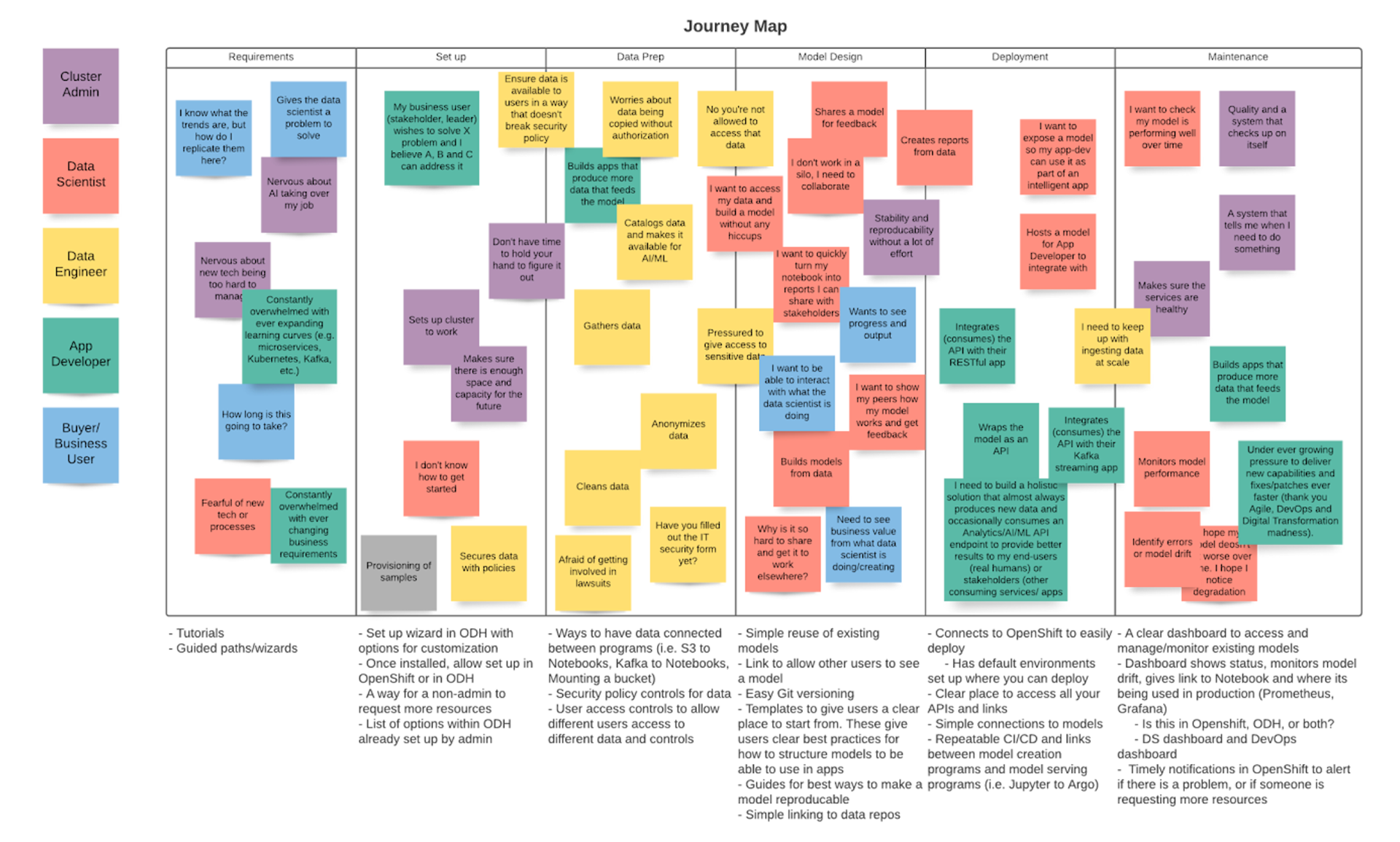
These weekly meetings became an important touchpoint for the team as the product grew. It served as the first place where all team members on the small team could discuss problem solving and understand the full end-to-end experience as we designed the product and got closer to a technology preview that would be released at Red Hat Summit.
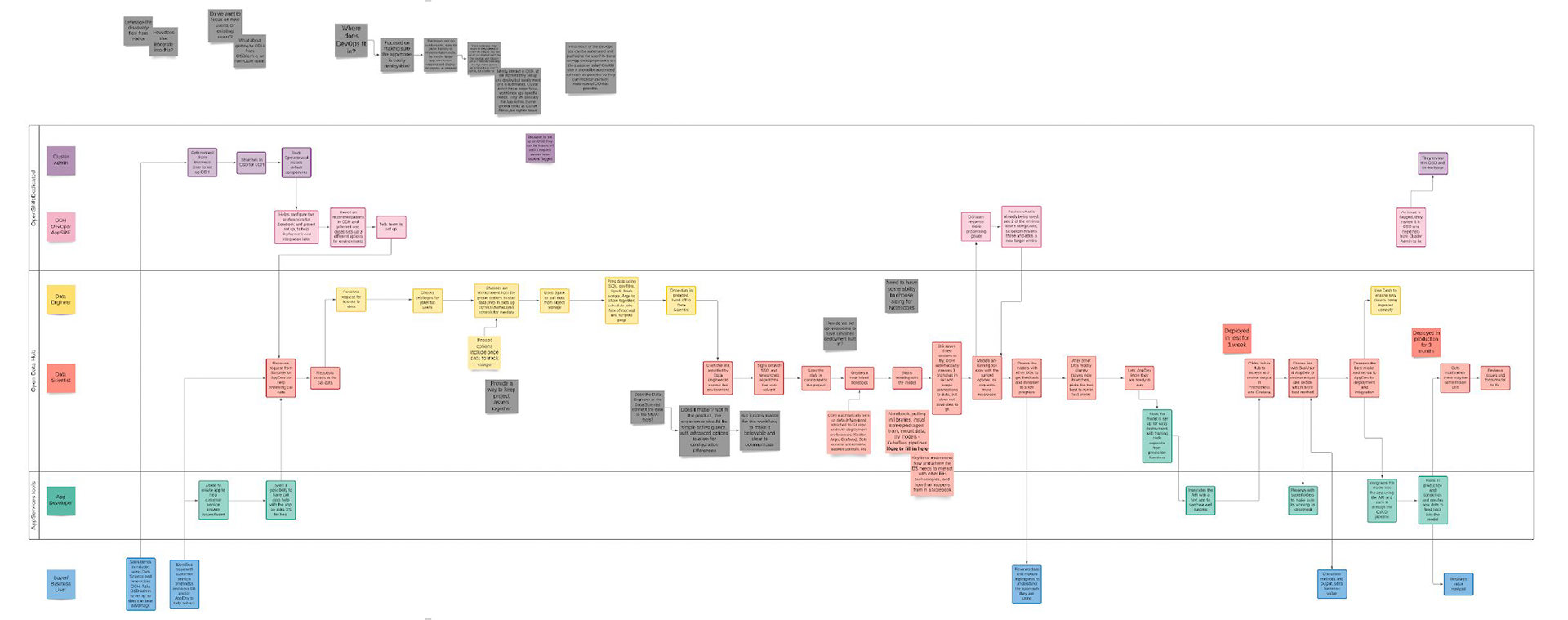
First Concepts
Once we had a clear view of their needs and the journey users needed to take, I started designing the pages we decided were the most important for the users.
There was an existing pattern for installing the product, but we wanted to augment that by allowing the installer to choose options for what users would see, and how the product would behave.
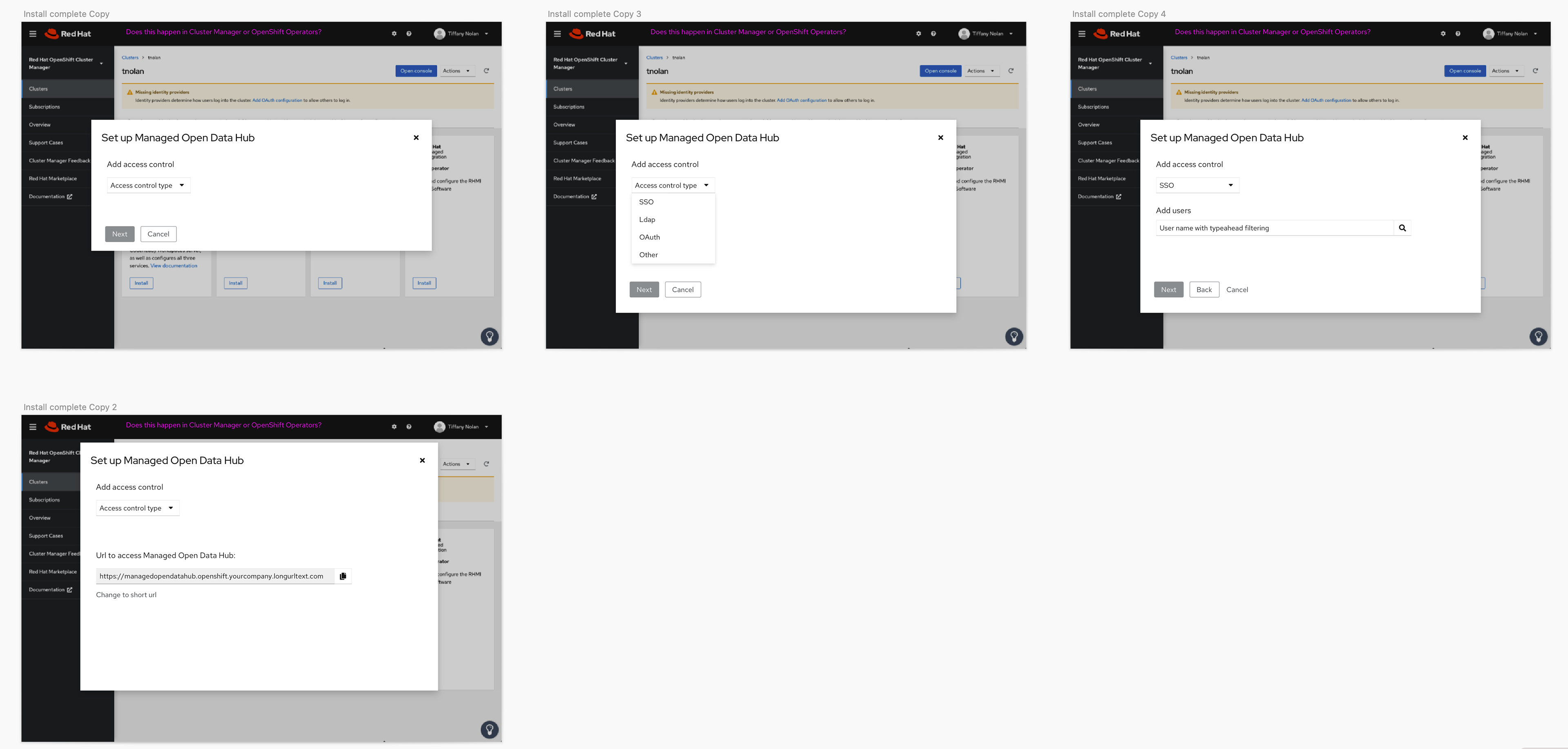
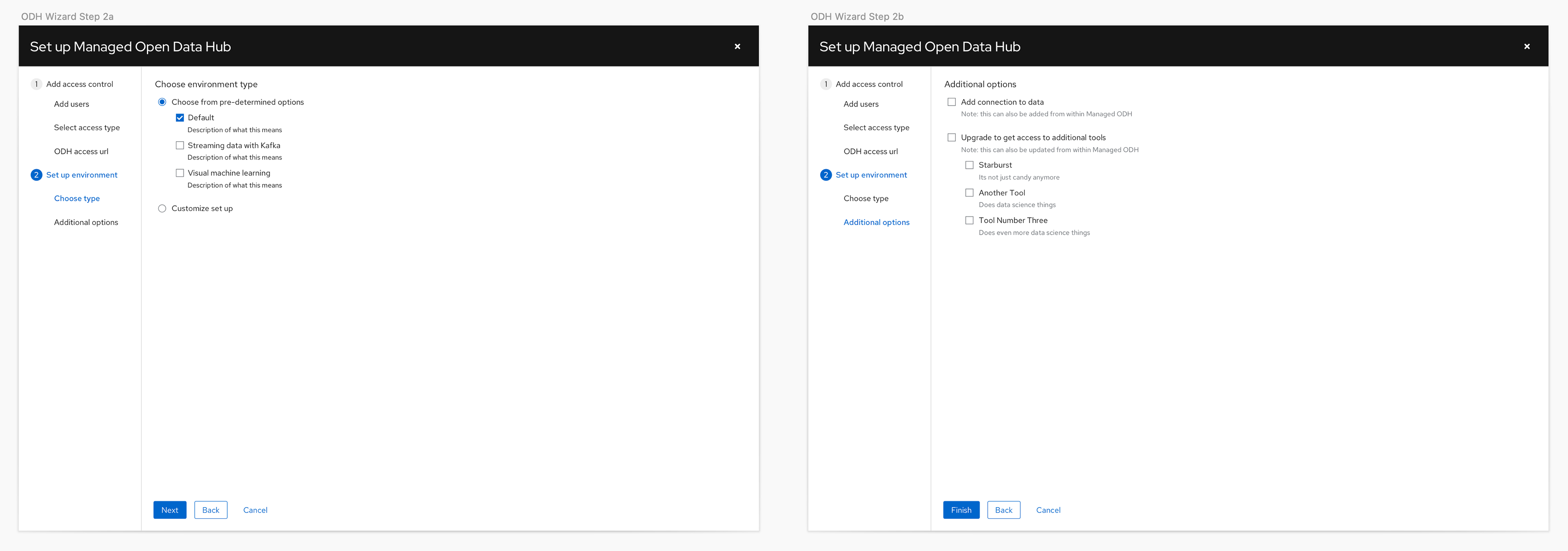
In the product itself we knew we needed a UI that would allow the users to access the different tools offered in the product, and learning content to help them get the most out of the features. We also wanted to create a better experience for launching Jupyter notebooks and a dashboard page to provide users and administrators one place to see status for the product.
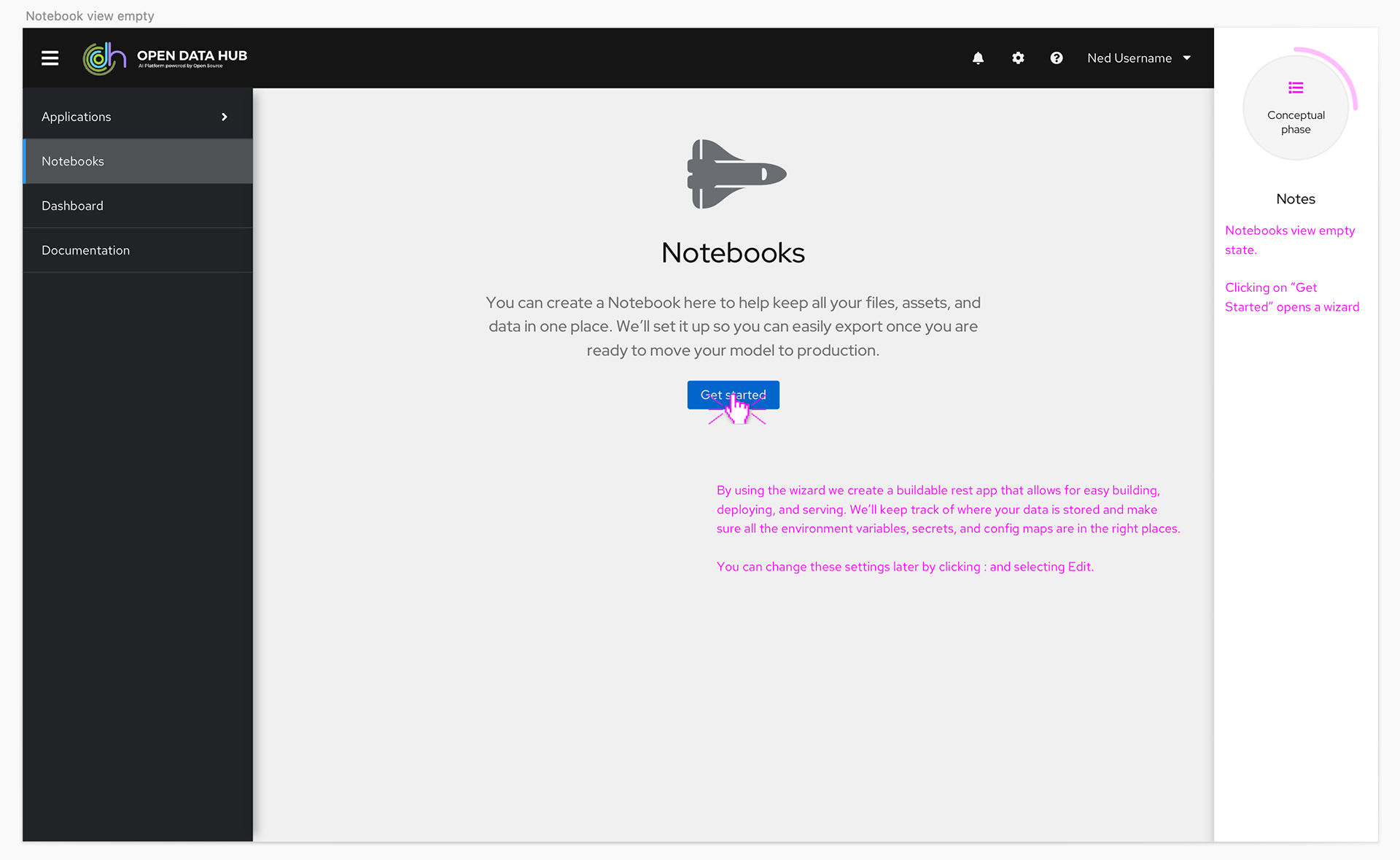
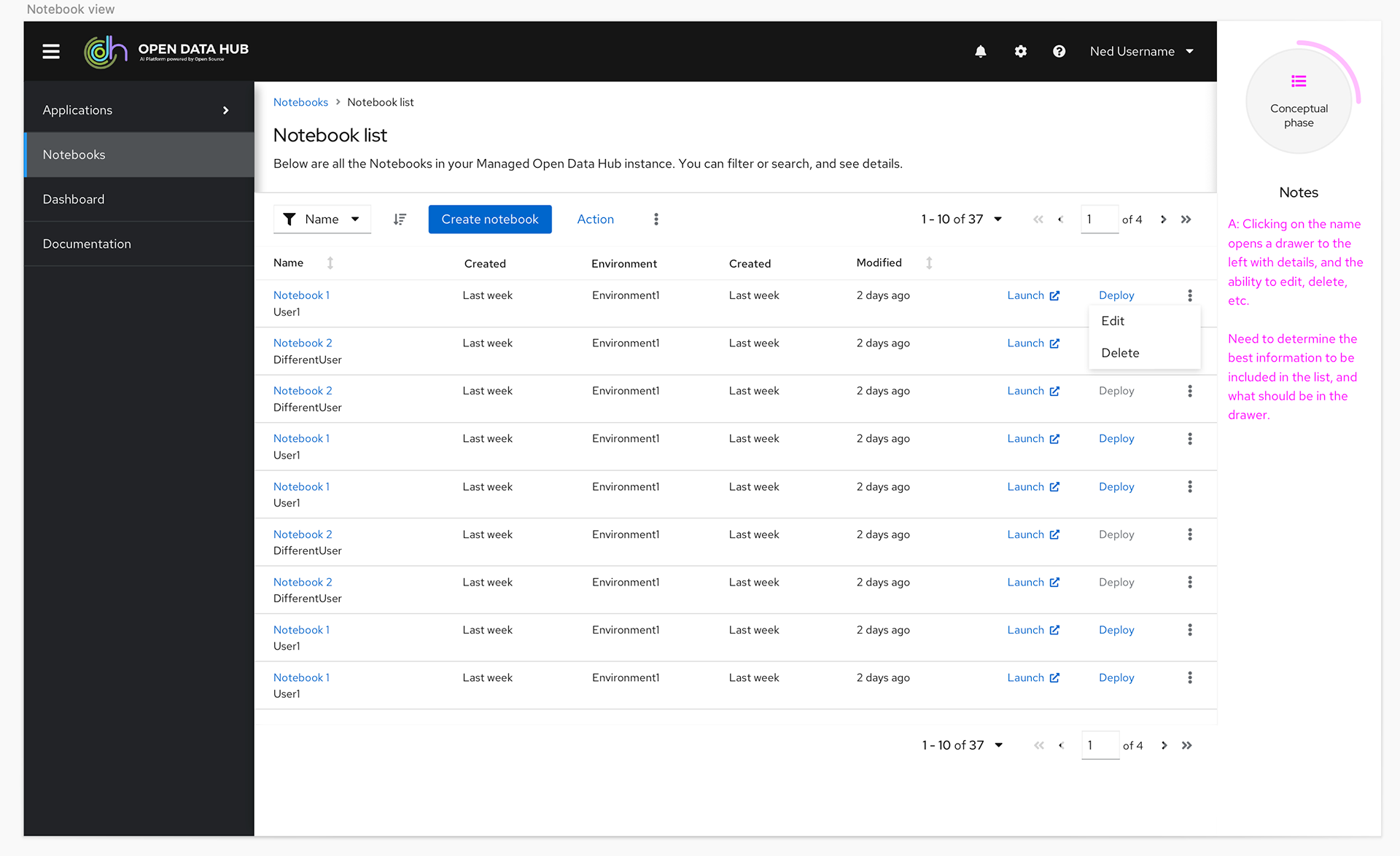
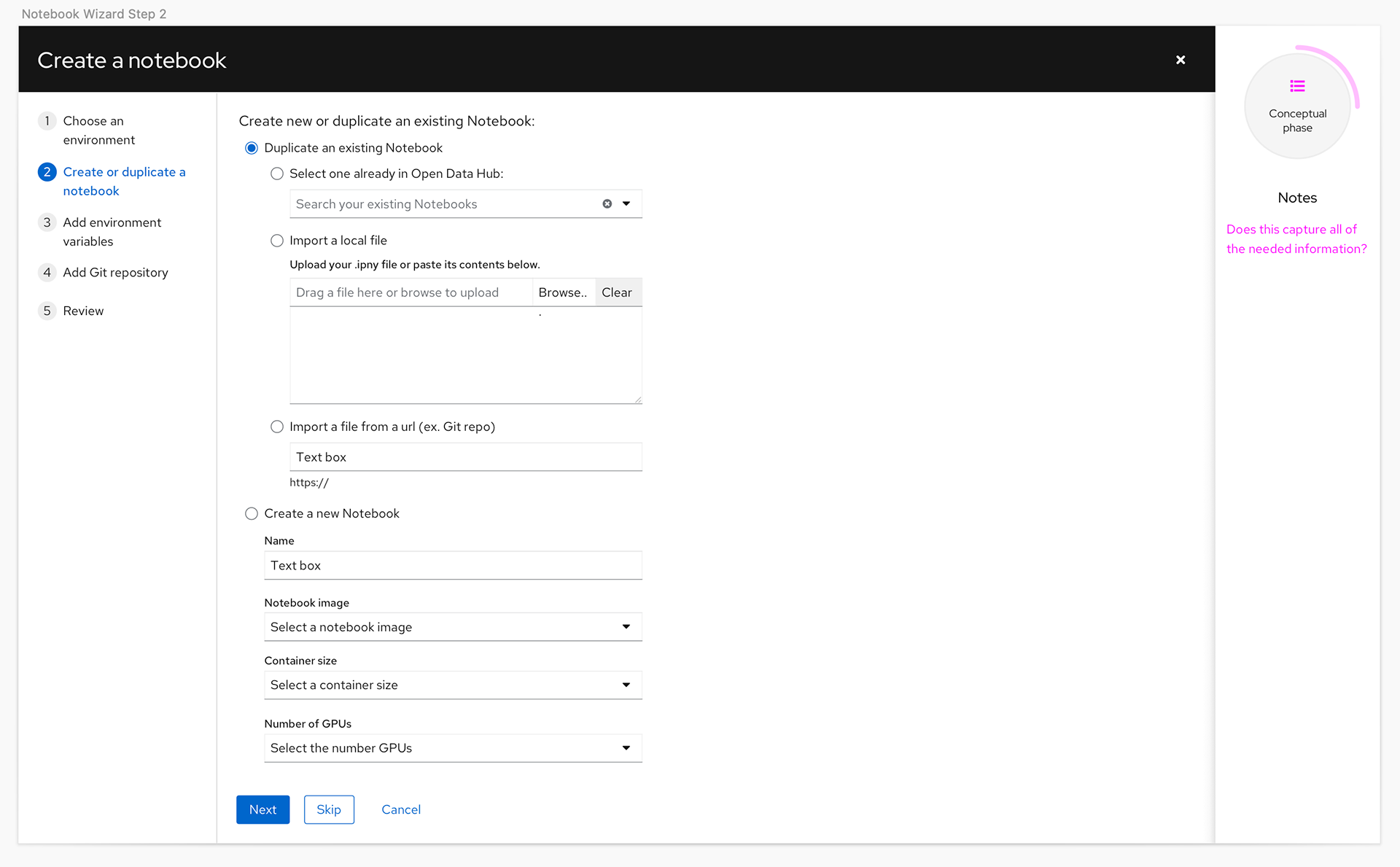
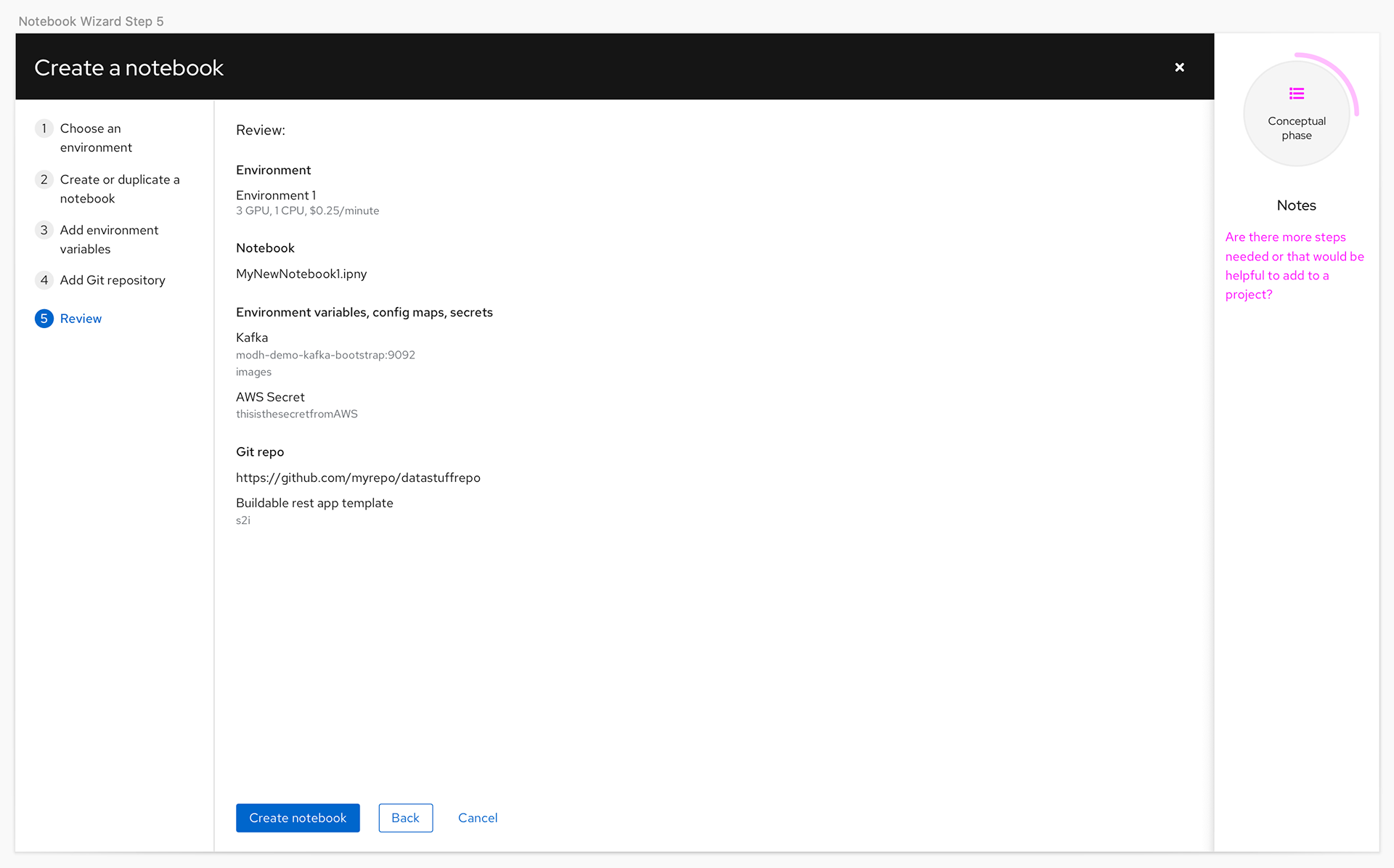
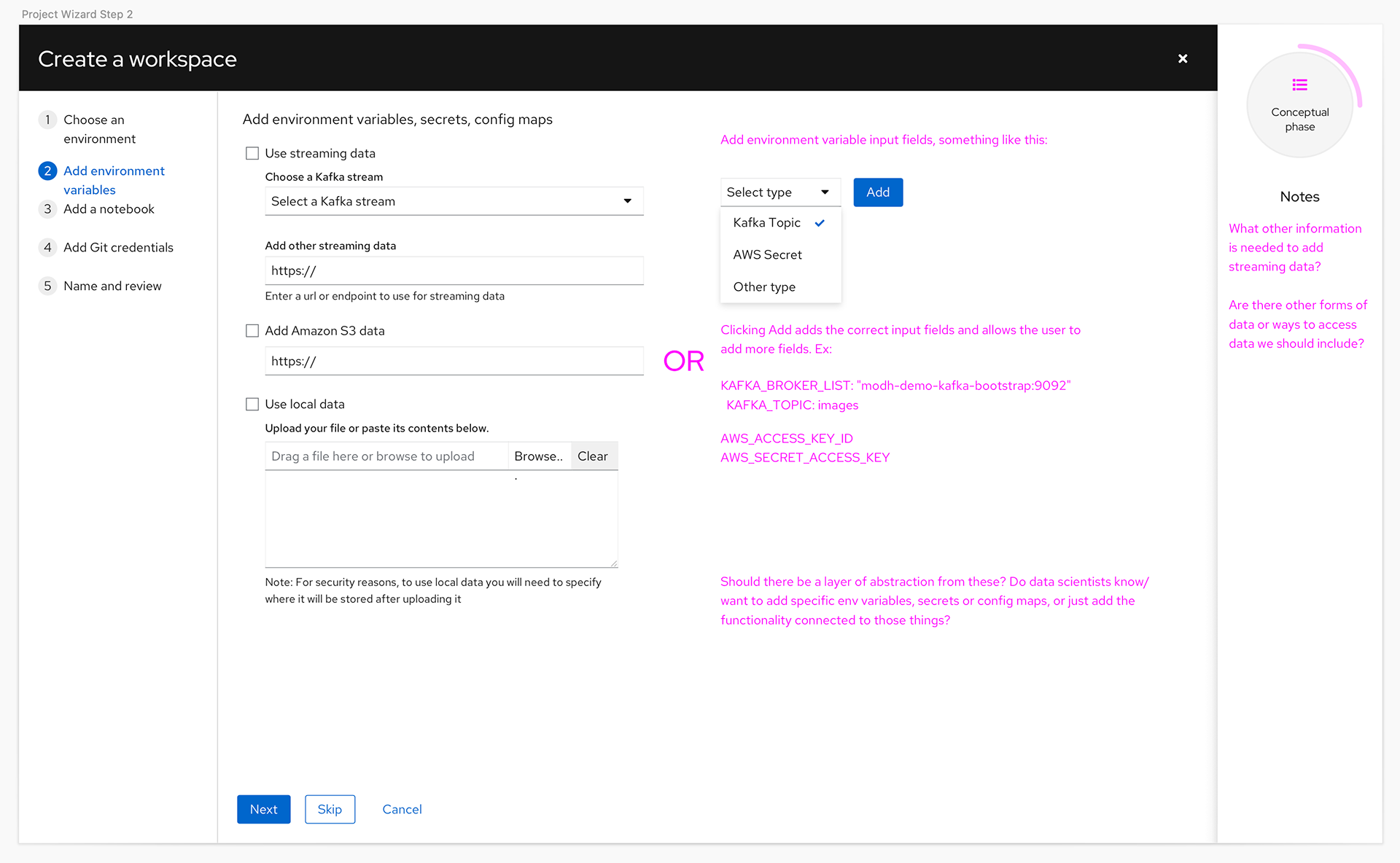
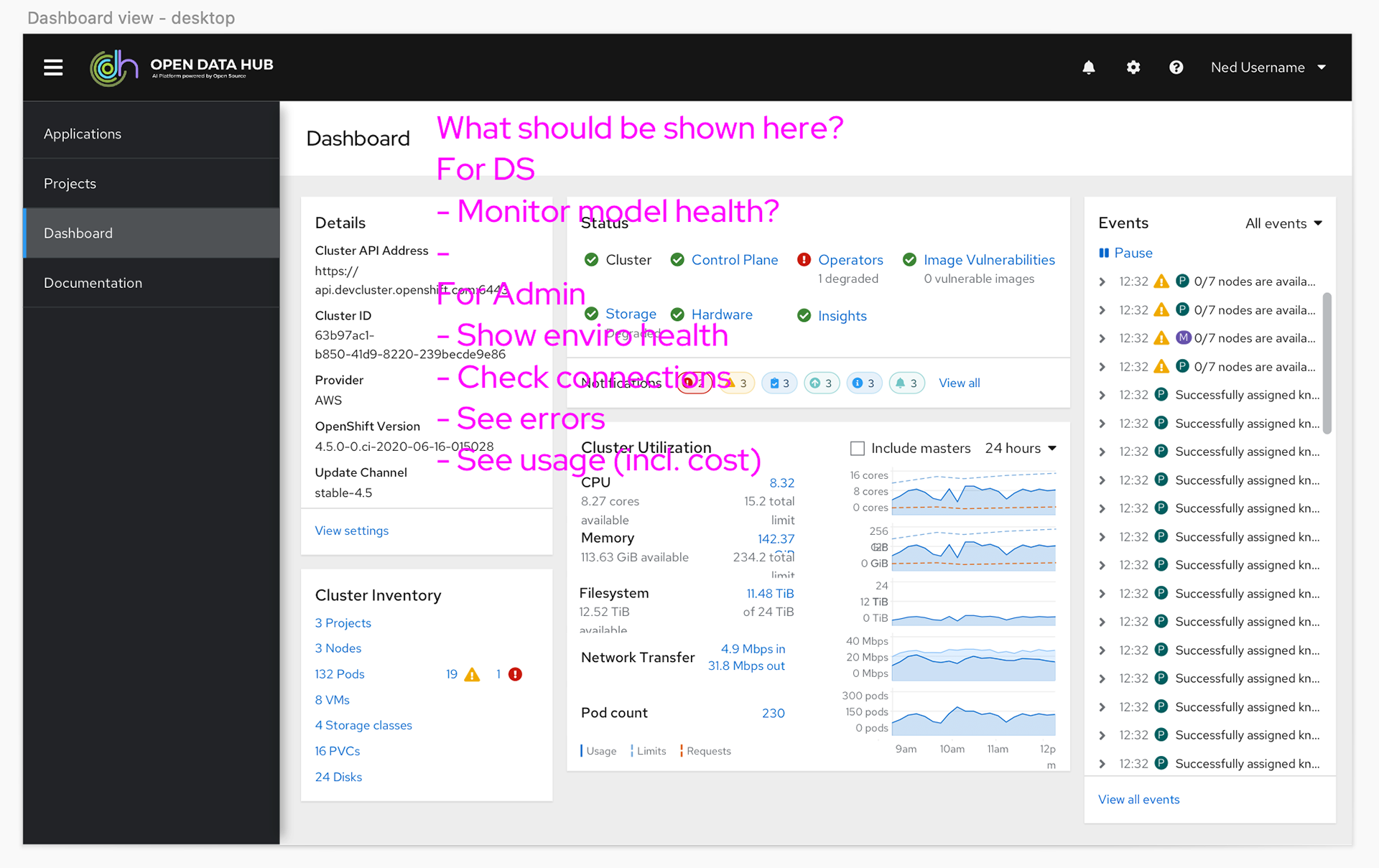
Re-scoping the MVP
After some exploration of the augmented installation flow and the notebooks the team needed to rethink the amount of work that would have to be completed with the small team. Once a more detailed review of the technical challenges for implementation was done the team determined it was too much work for the team size and the planned GA date. For the technical preview and GA we decided to focus on what would be the Application pages and learning, and relied on an existing pattern for the installation.
Final pages
Using Patternfly, Red Hat's design system, I started to build pages with existing components to make sure it would be easy to implement and understand the options available. It quickly became clear a card view was the best way to display the applications, so to give the users a consistent experience we designed the documentation page with the same pattern.
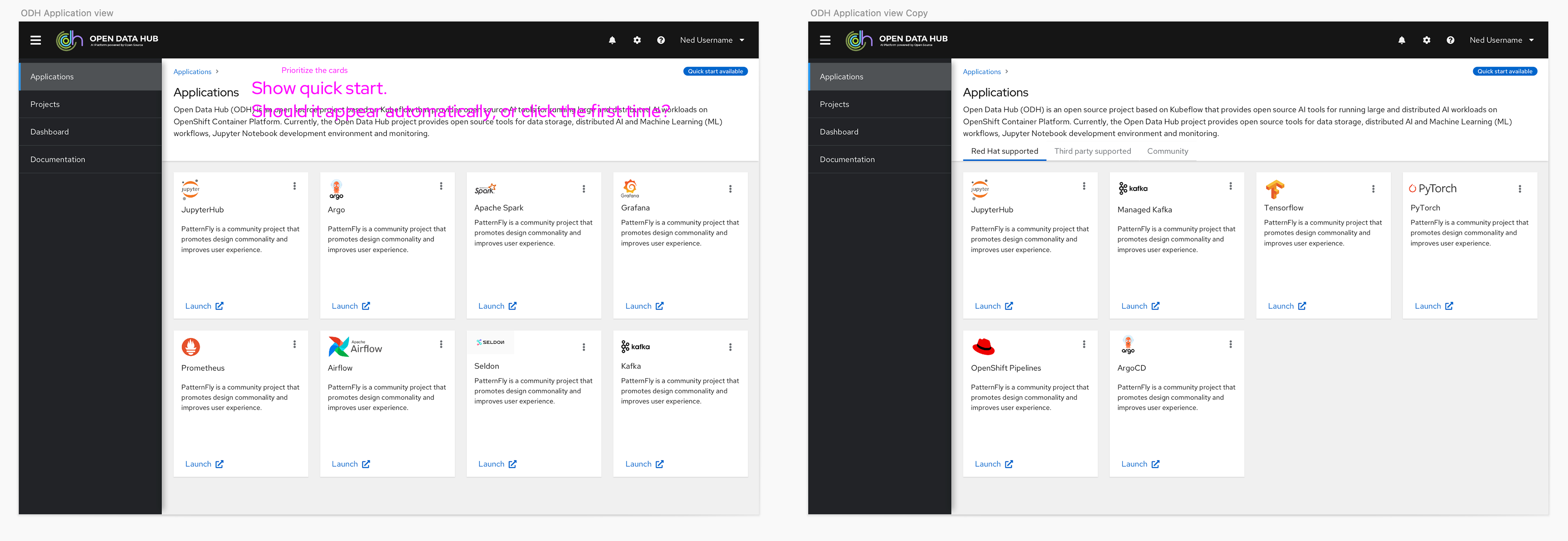
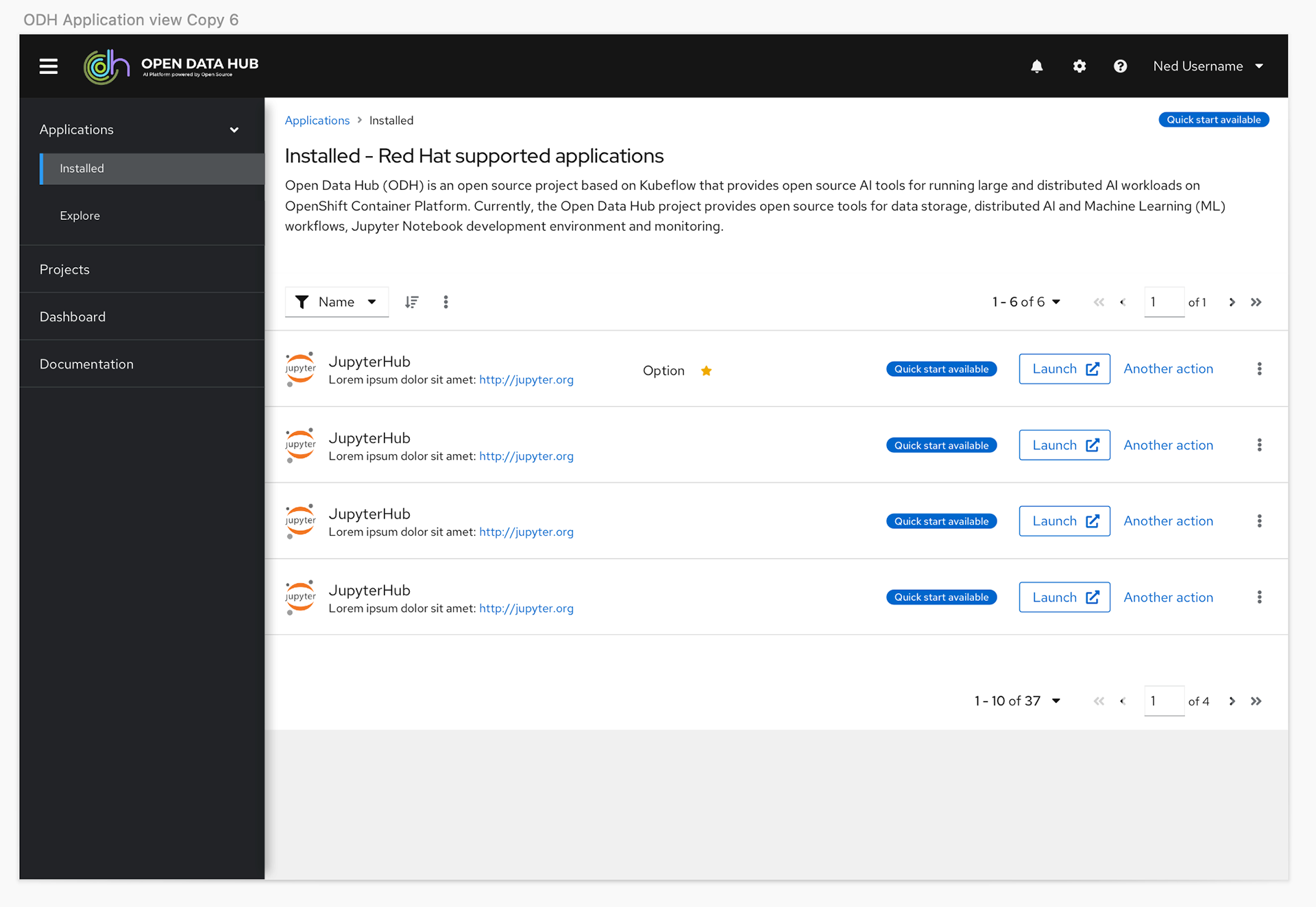
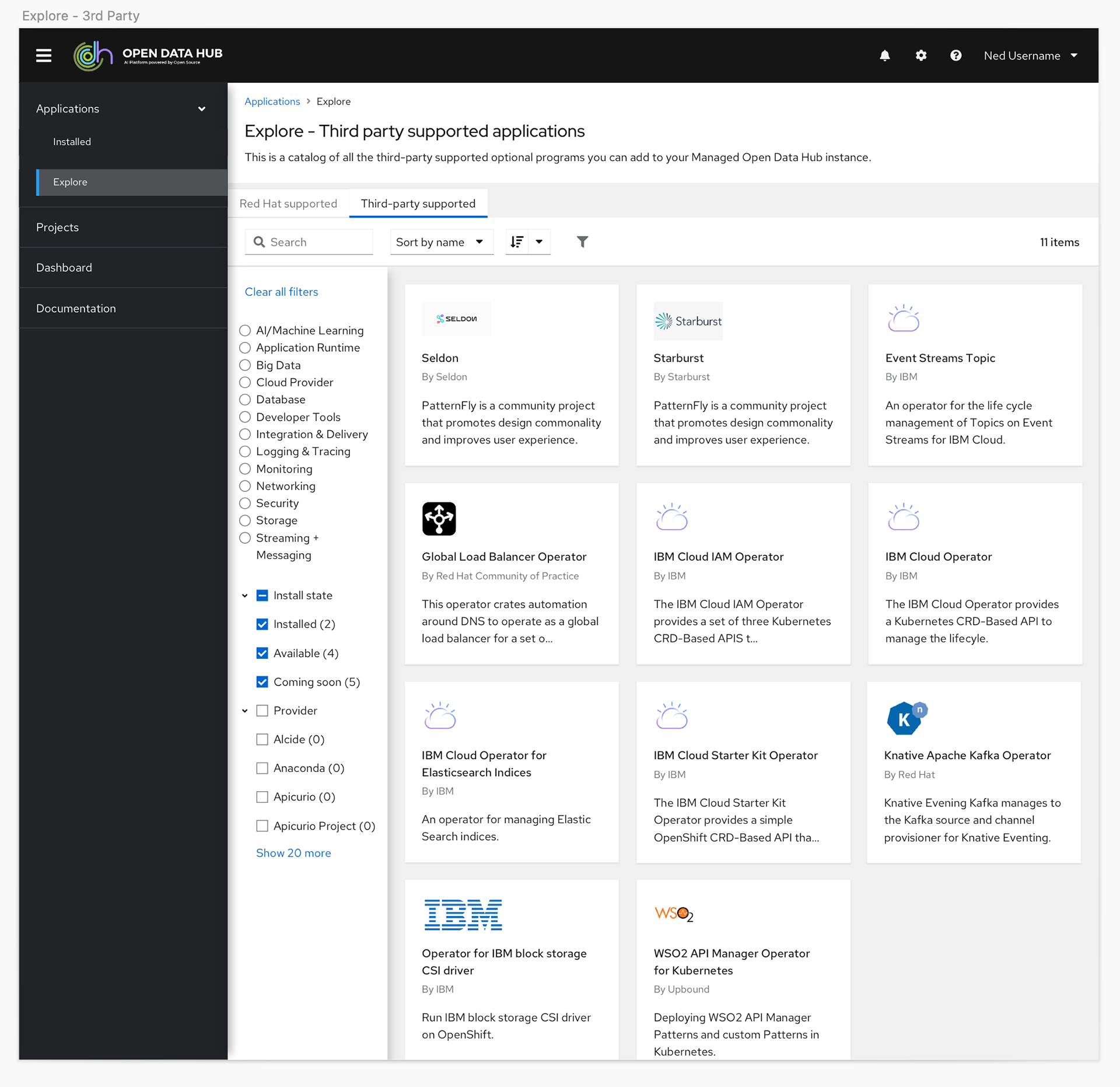
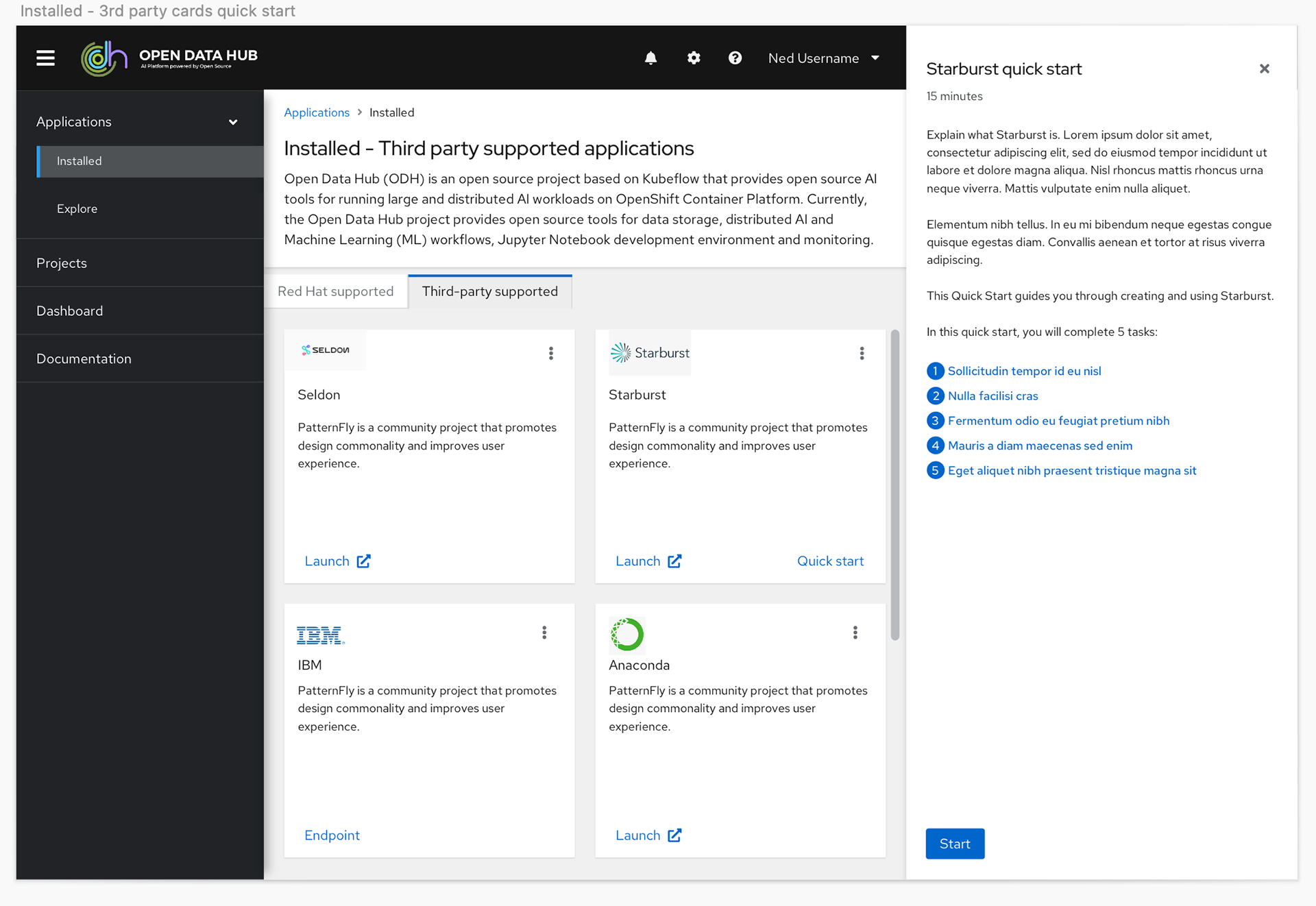
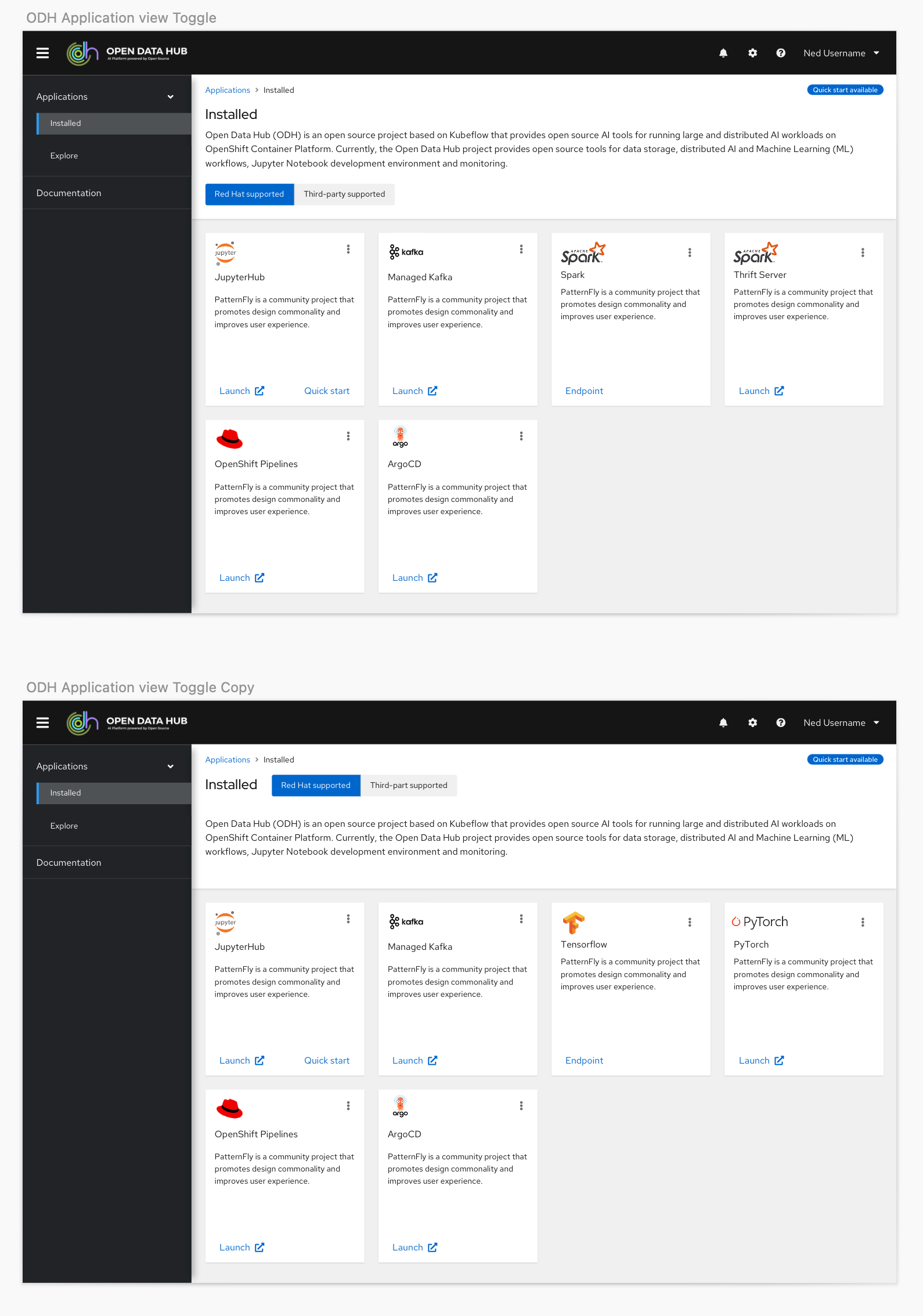
We originally had simple filters designed into what became the Resources page to help users find the content that was most relevant to them. However, given we were working with partners who had the ability to add their own content and we had four different types of content, when we got closer to the final GA date we discovered there would be more than 40 unique items users would have to sift through to find the content they needed.
With this knowledge we decided to shift the plan to give users more granular control with a catalog view, and to also give them the option to toggle between a card or a list view so they could see more options on a page at a time.
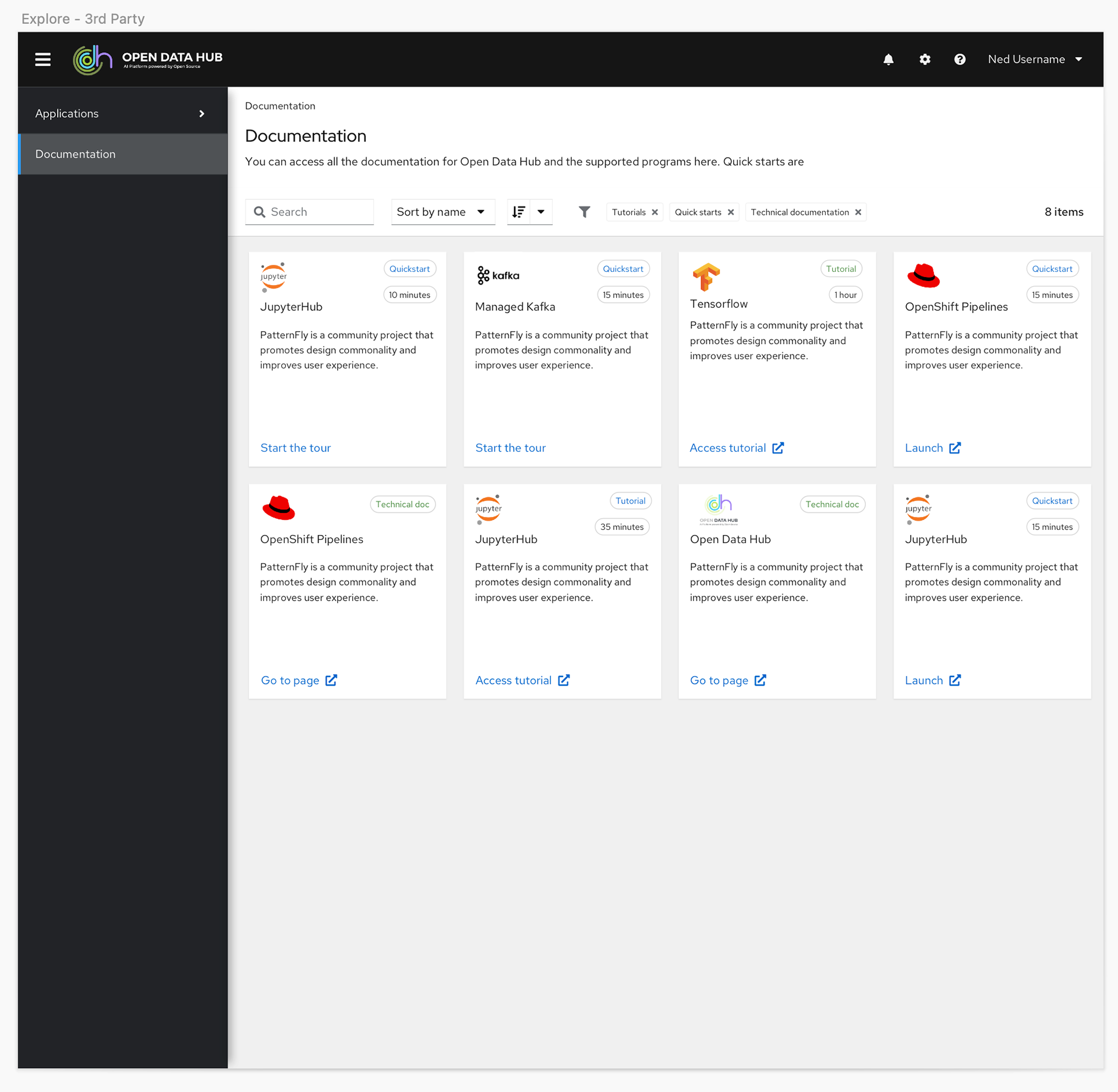
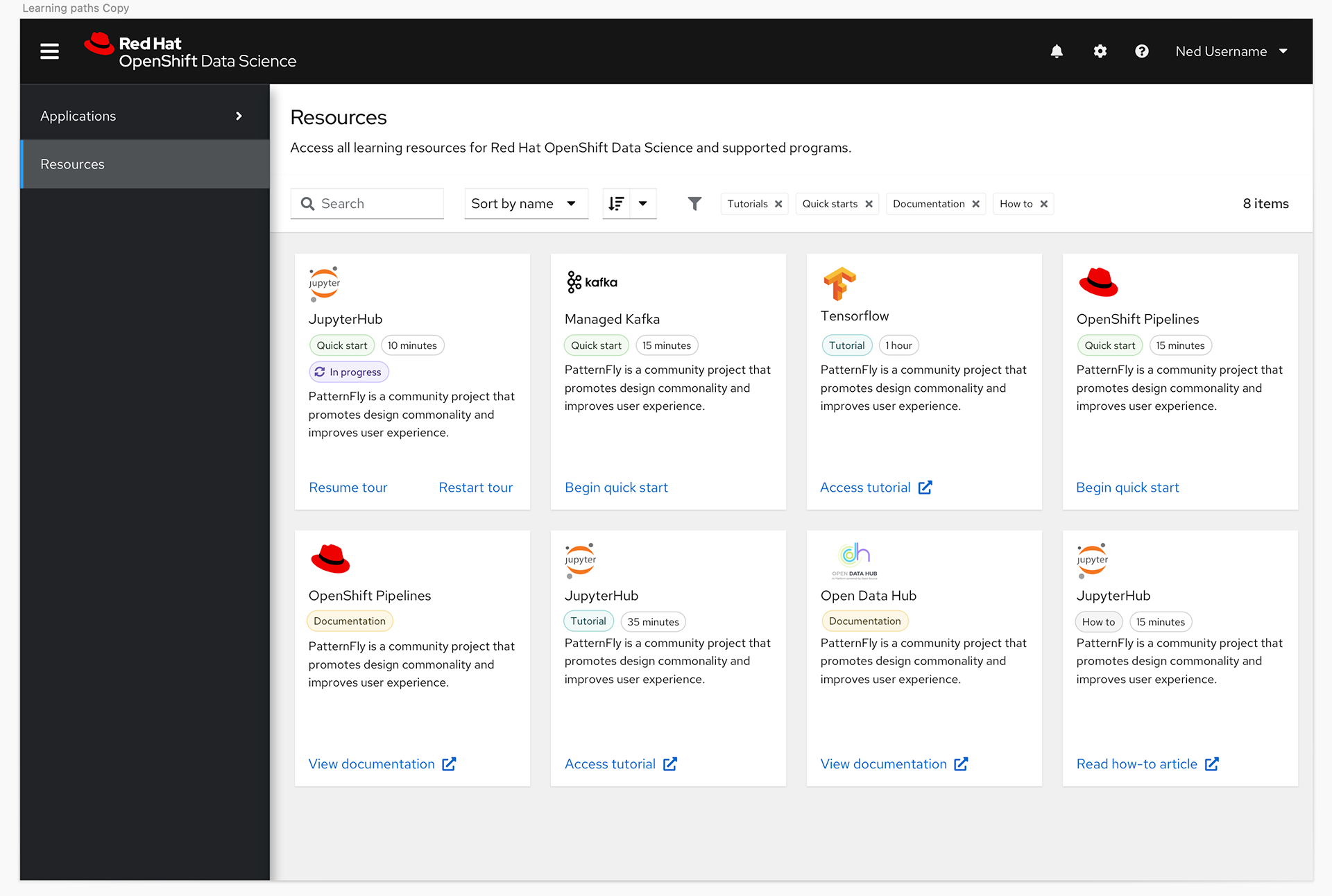
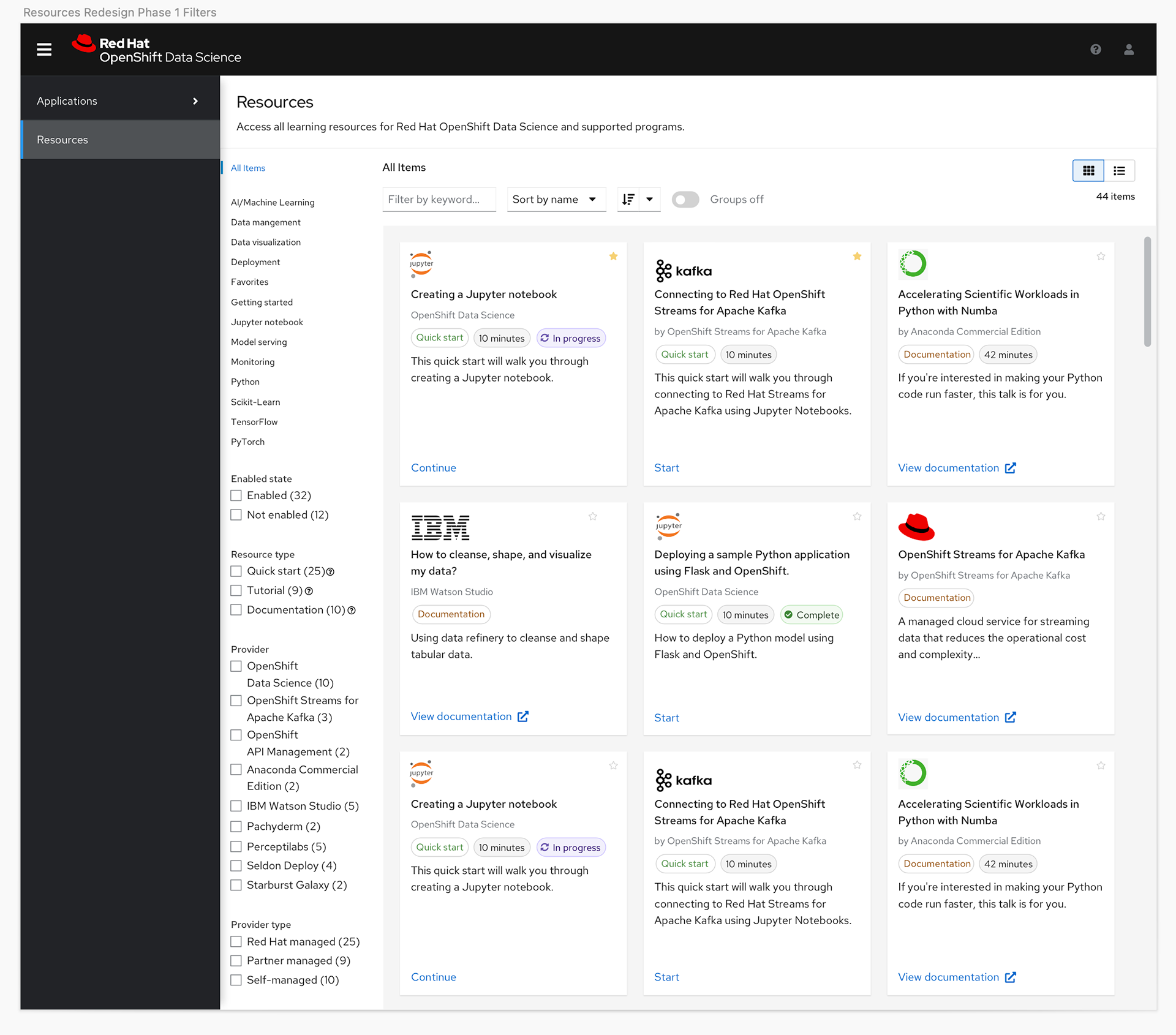
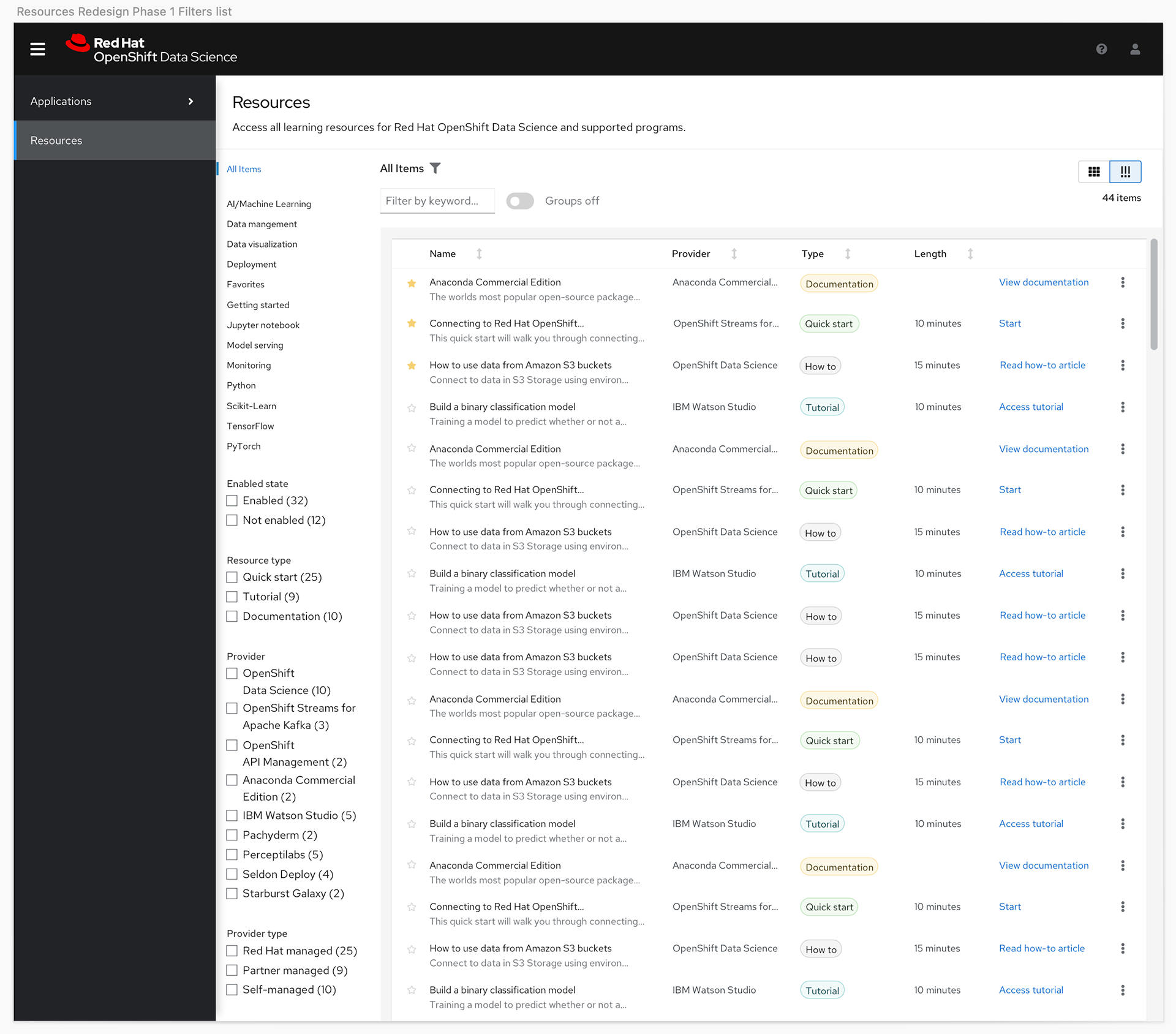
Tech Preview and Next Steps
The product's Tech Preview was well received as Red Hat's first foray into the data science and AI/ML platform, but it was immediately clear we needed to expand the product to add the functionality that was removed from the MVP.
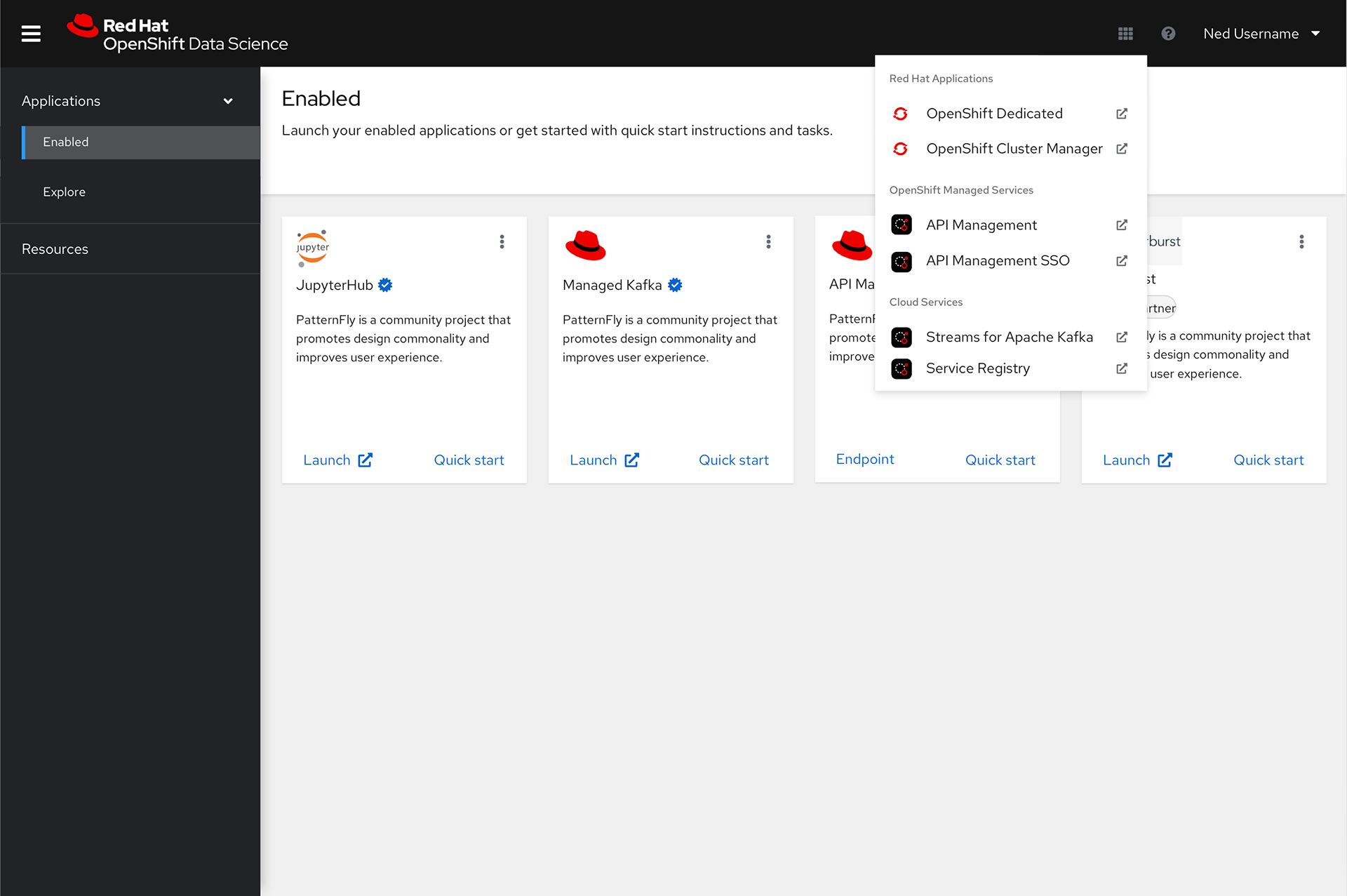
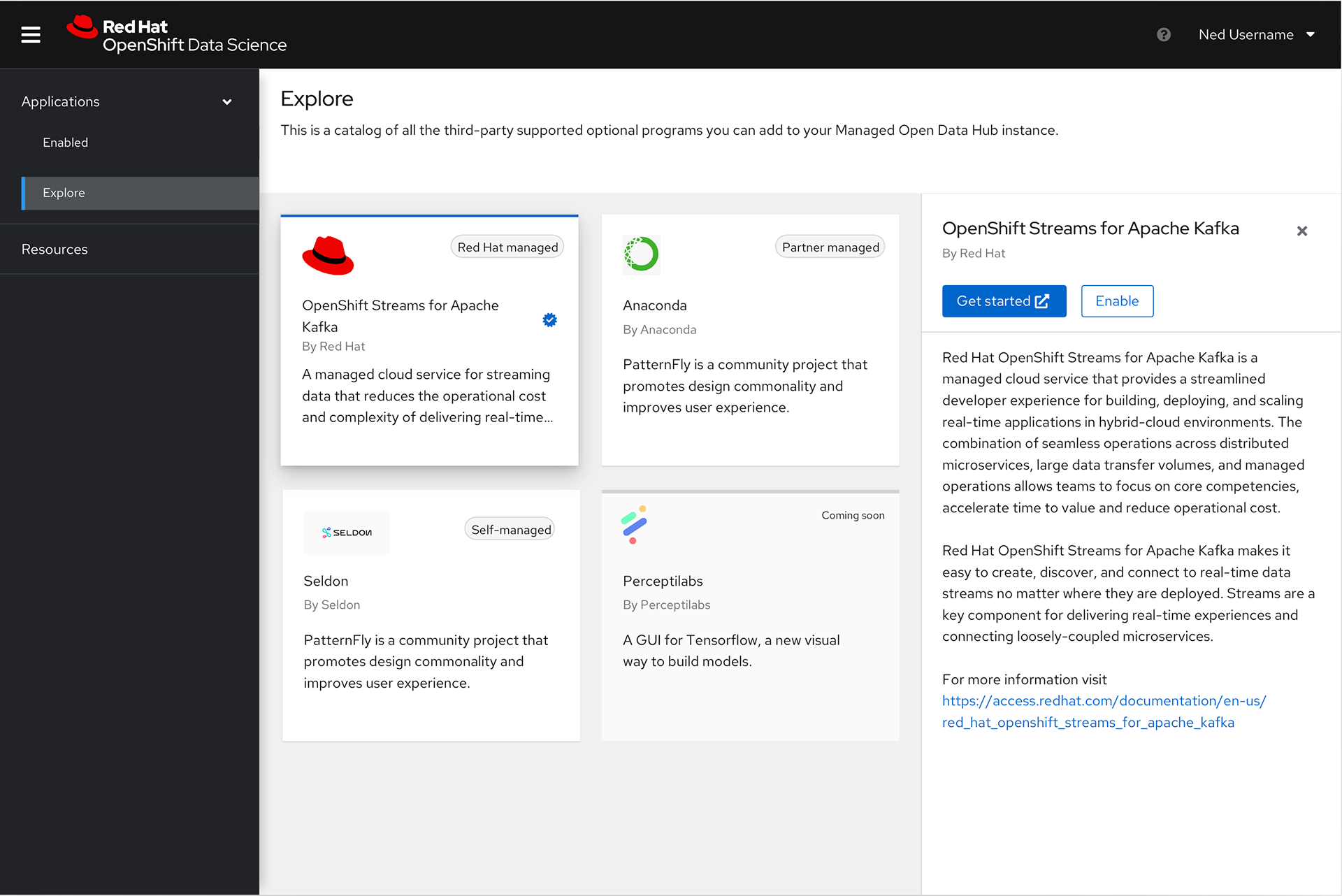